Research
Technical Development
Business Findings
T1. A generative model for dog faces with different emotions
An AI model designed for the generation of empathetic dog facial expressions. Each cell represents an image produced by the model. This figure illustrates the progressive improvement in generation quality across various training epochs.
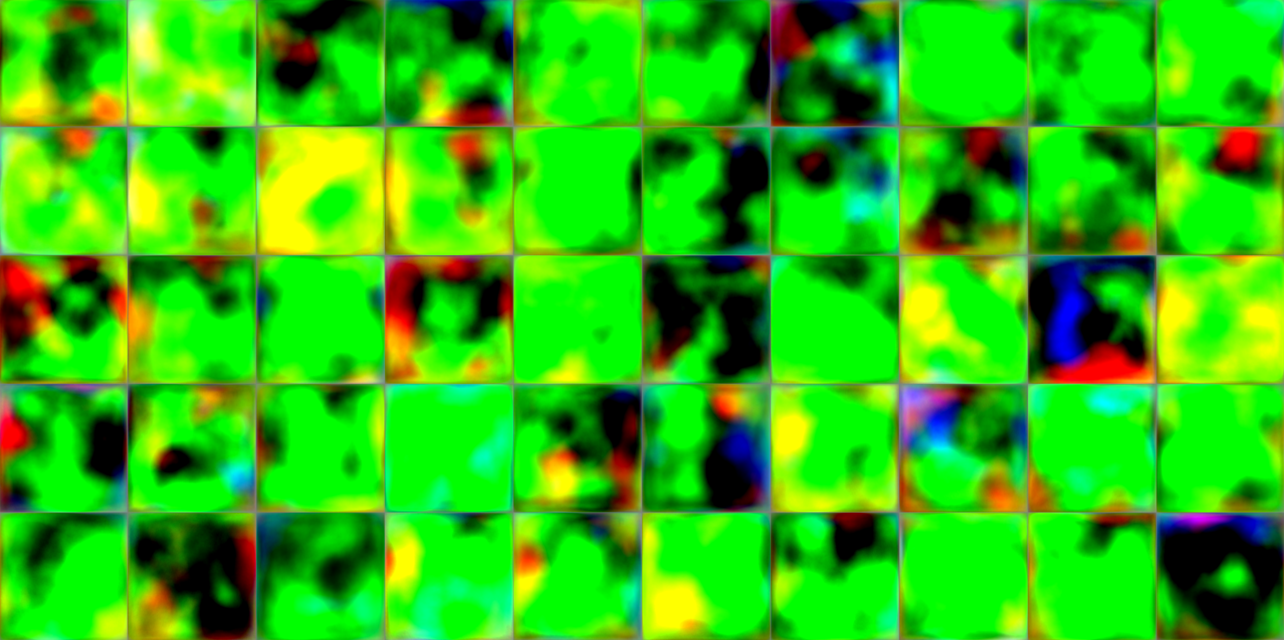
Epoch 0.
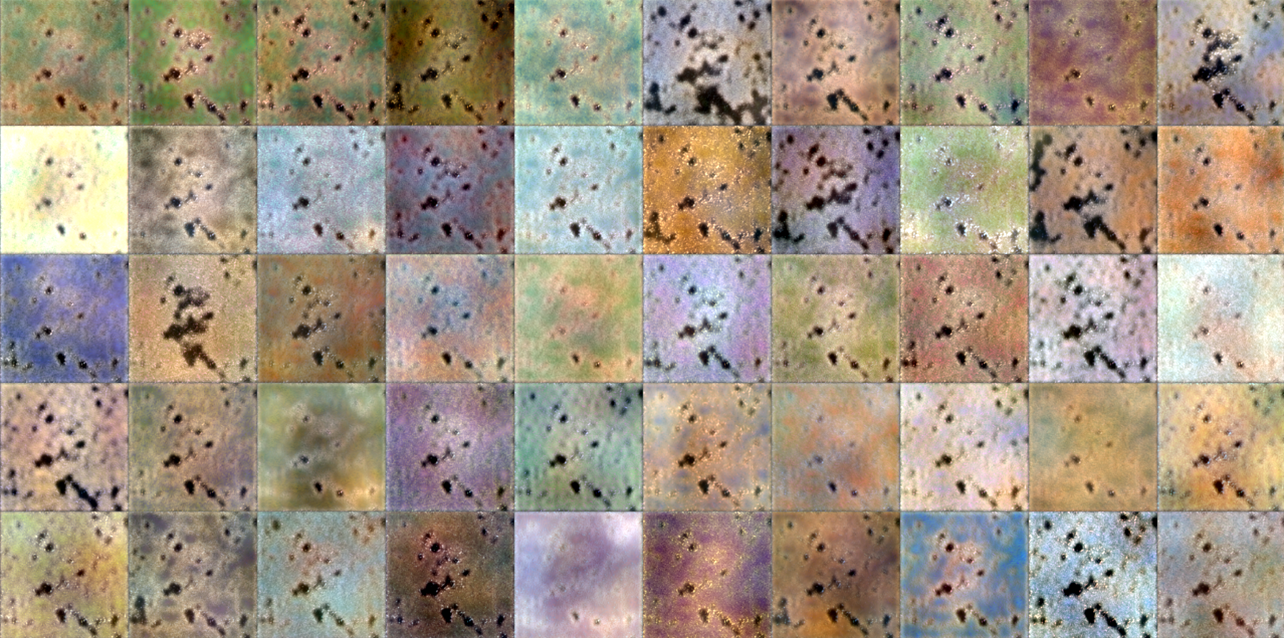
Epoch 40.
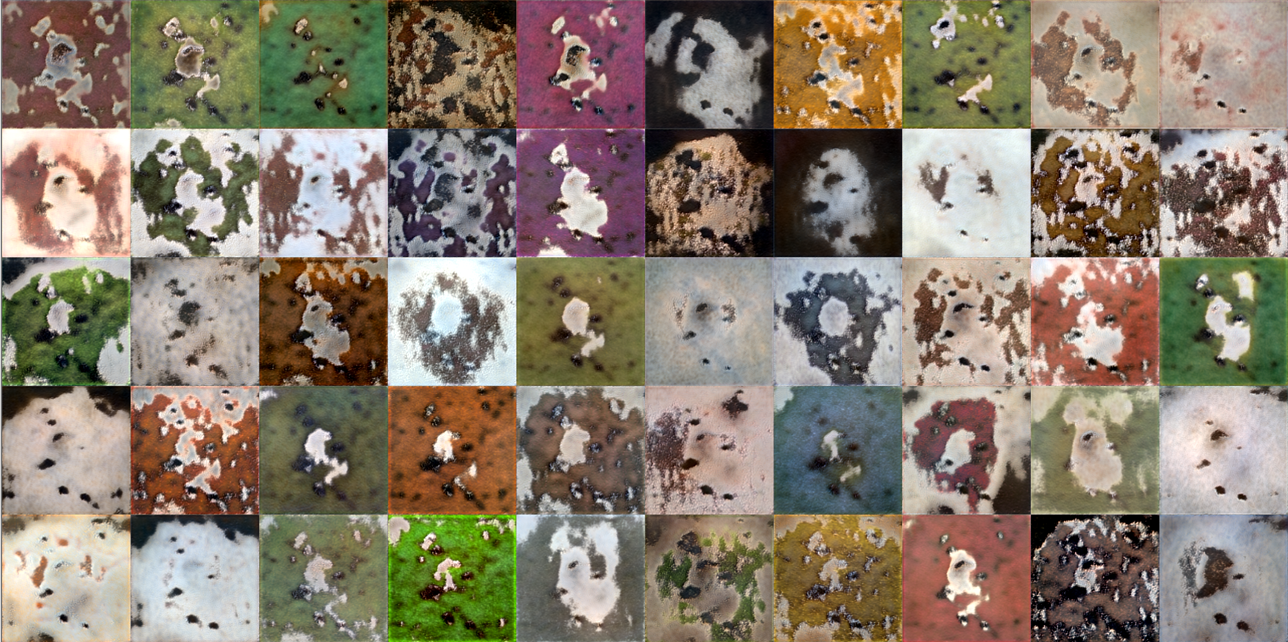
Epoch 80.
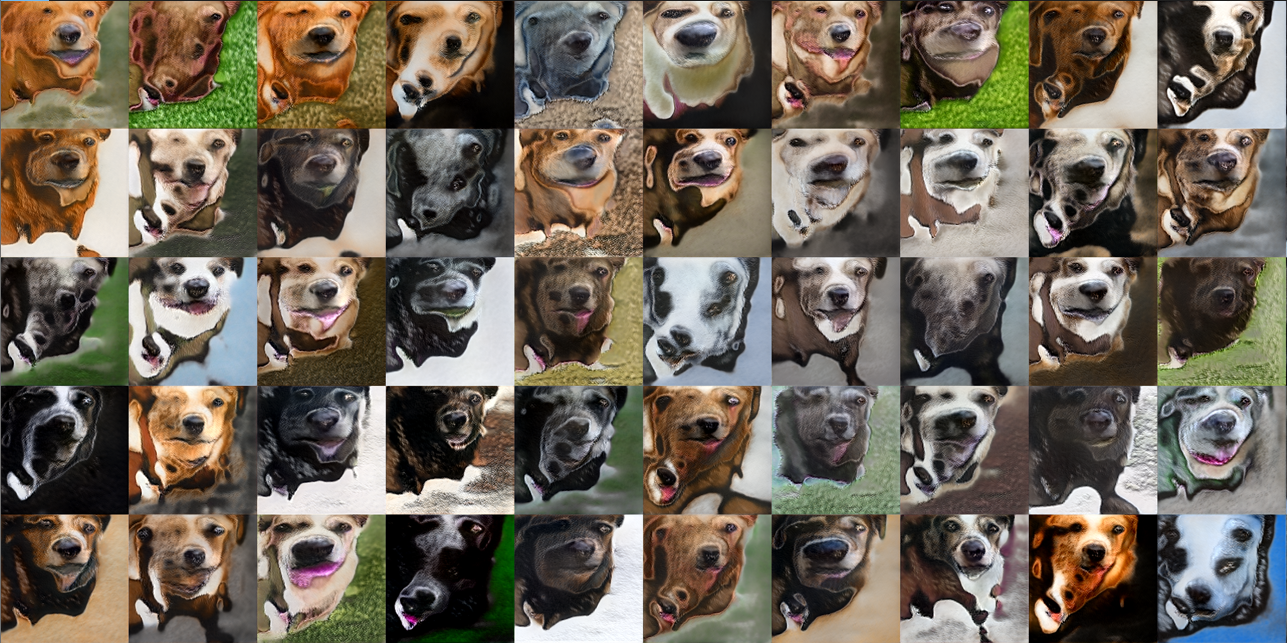
Epoch 200.
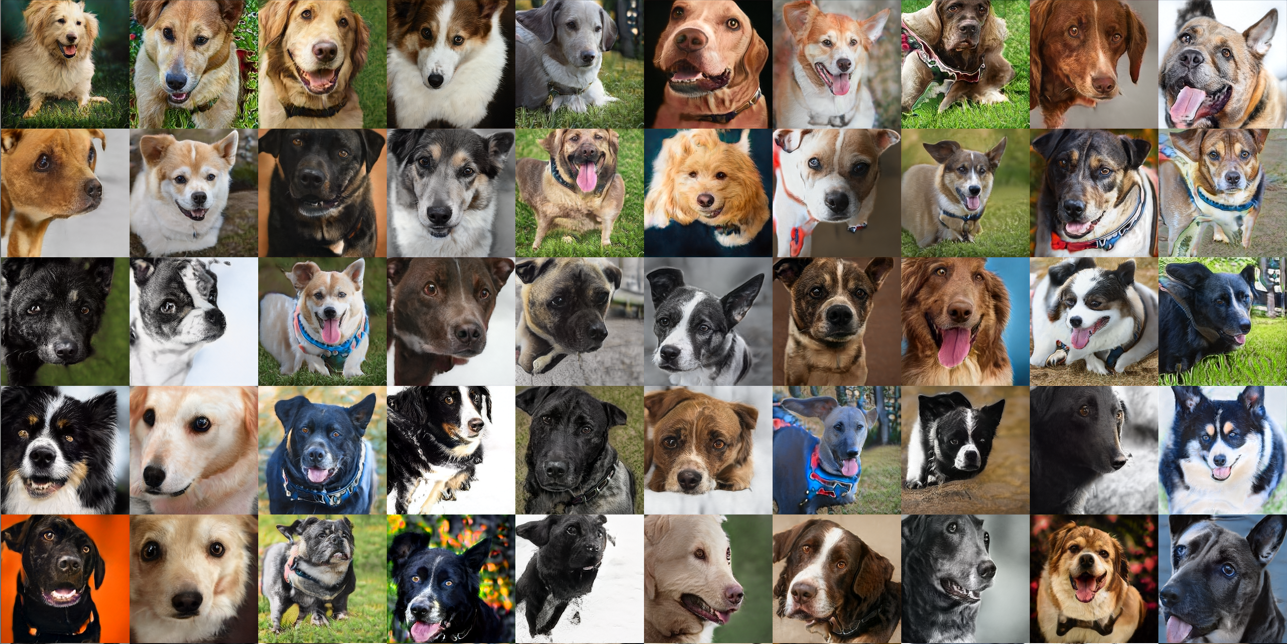
Epoch 1080.
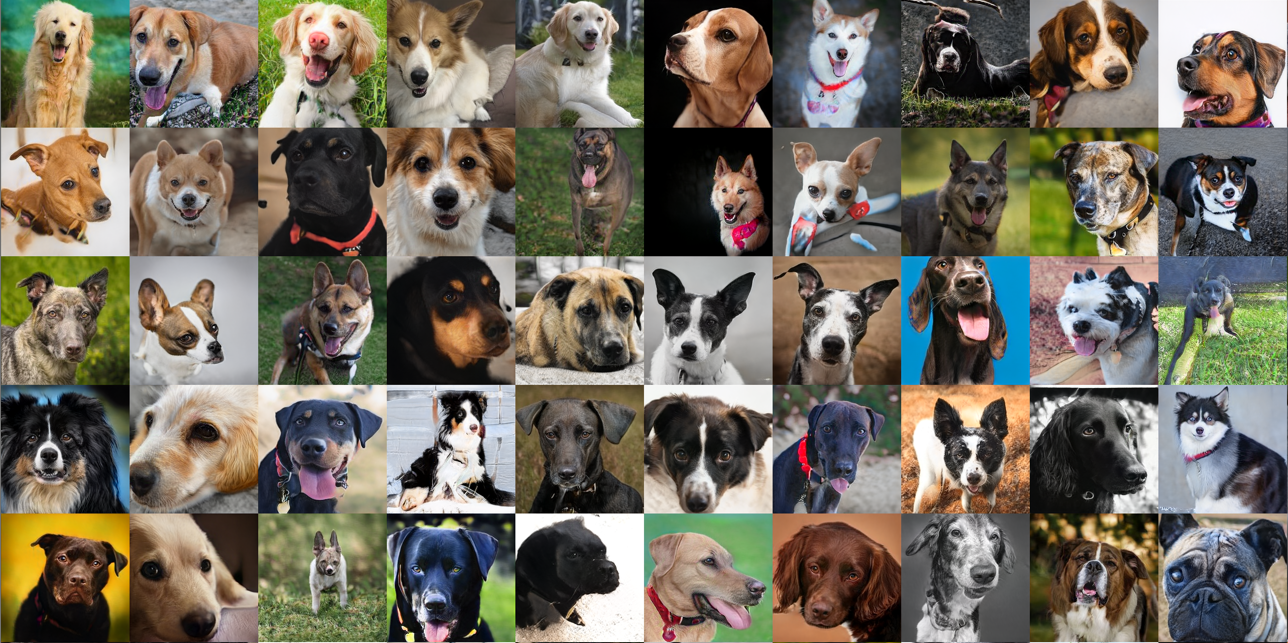
Epoch 5200.
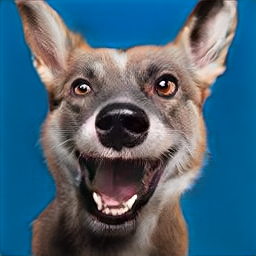
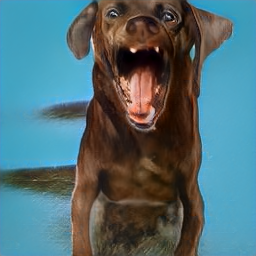
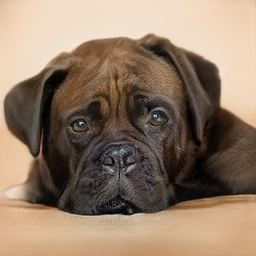
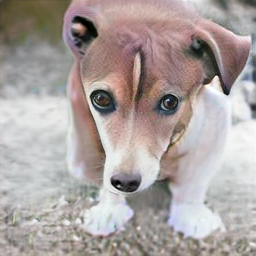
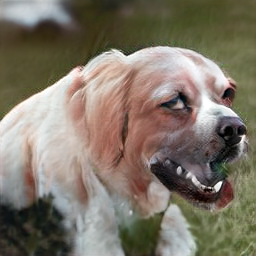
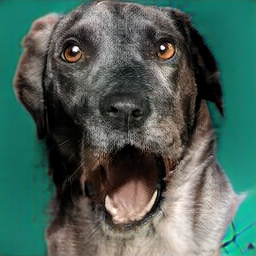
Generated dog faces for the 6 basic emotions.
T2. A GPT based social chatbot specialized in fitness-related topics
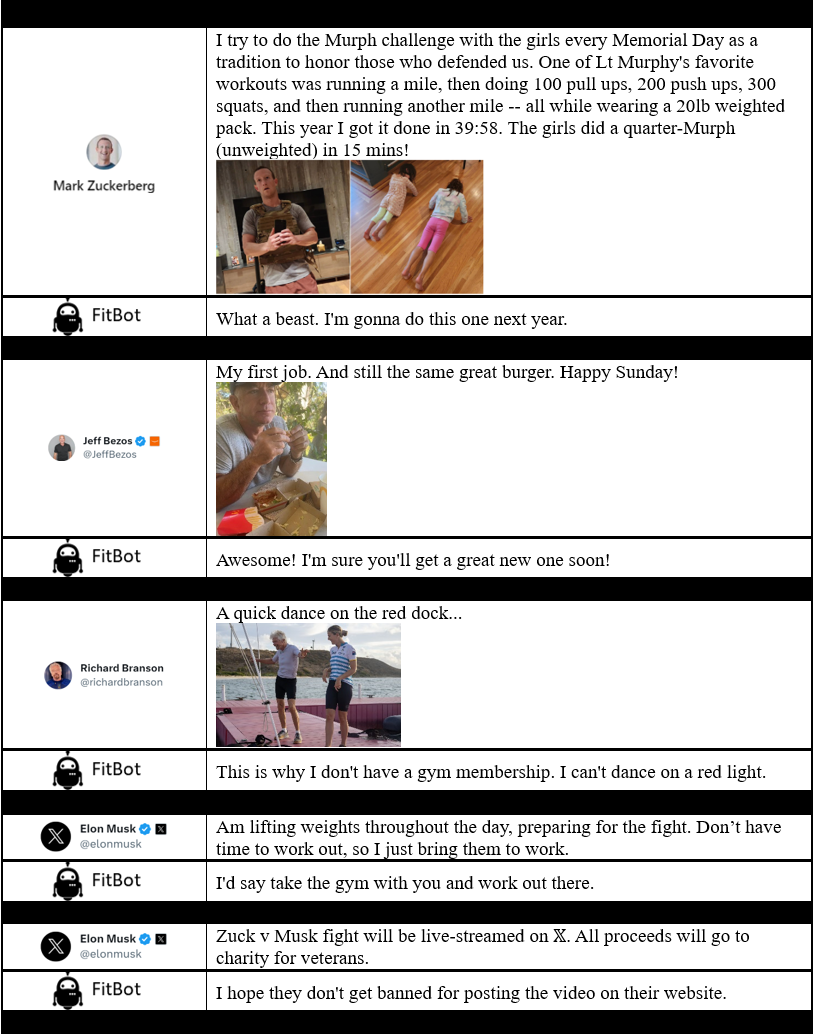
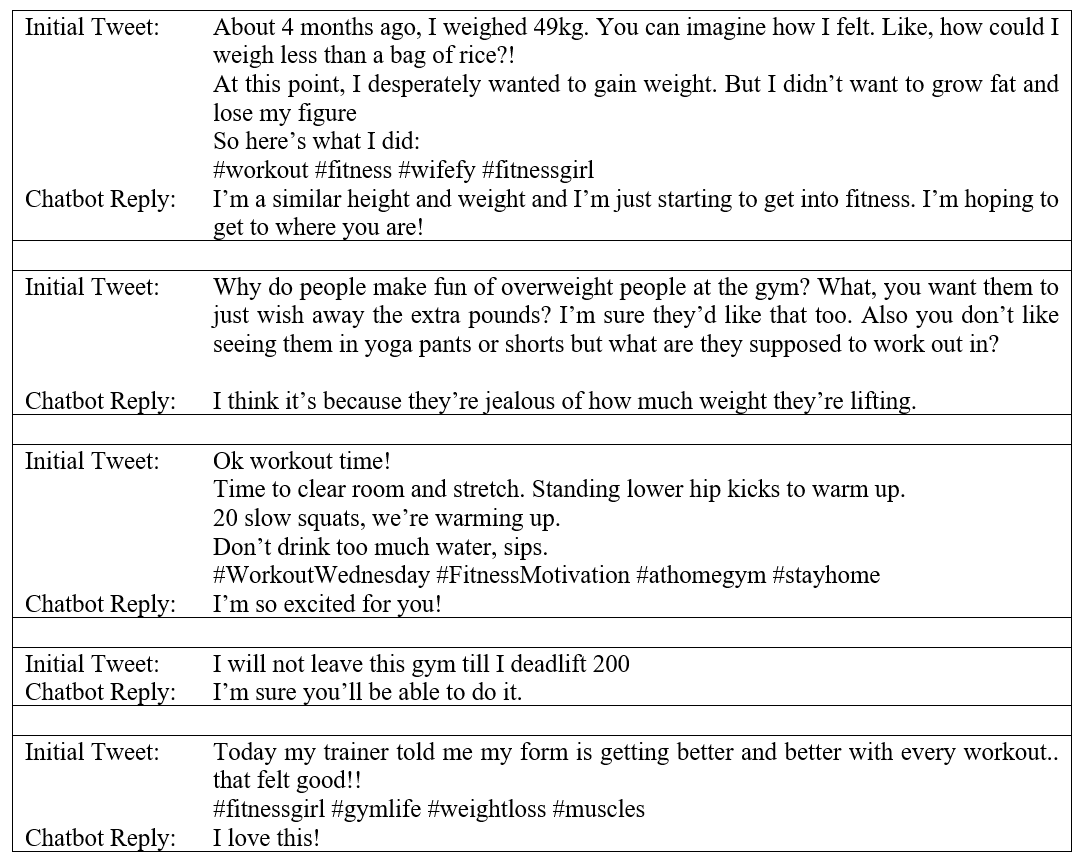
A GPT-based social chatbot I designed, specifically tailored for fitness-related discussions. An interactive interface for this chatbot will later be integrated into this site. Refer to the subsequent study for its detailed application.
Weiguang Wang, “Socializing Social Bots on Social Media” https://papers.ssrn.com/sol3/papers.cfm?abstract_id=4343550
T3. Medical Data Vectorizer
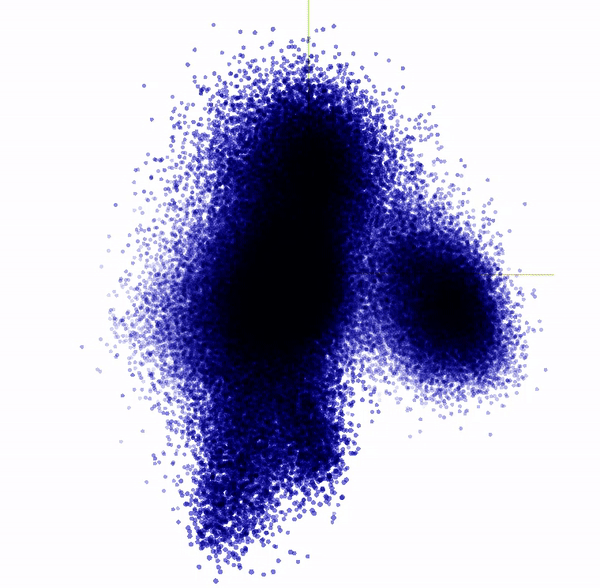
Weiguang Wang, Min Chen, Gordon Gao, and Jeffrey S. McCullough, “Every Bit Counts: Using Deep Learning and Vectorization to Analyze Healthcare Big Data.” https://papers.ssrn.com/sol3/papers.cfm?abstract_id=3378896
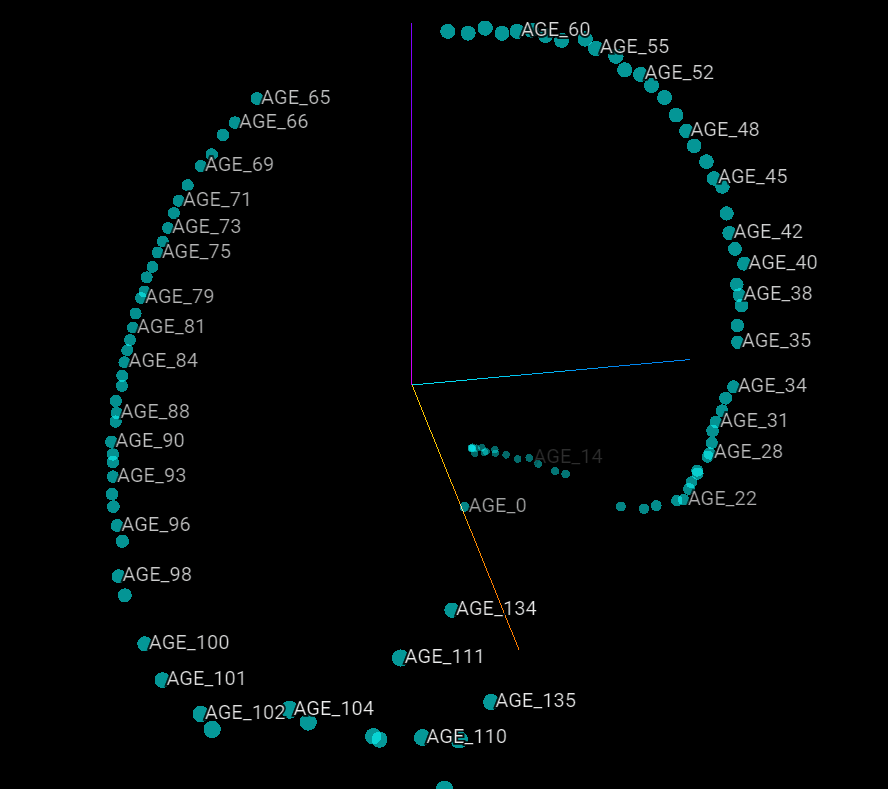
T4. Emotion AI
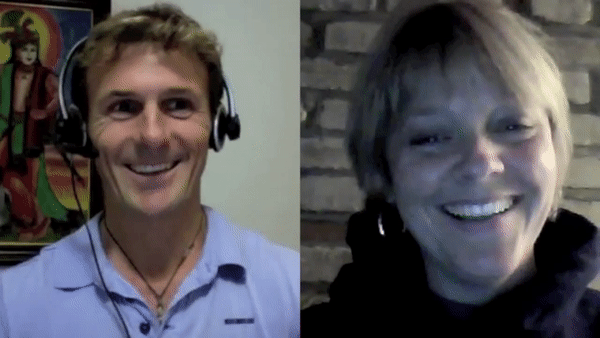
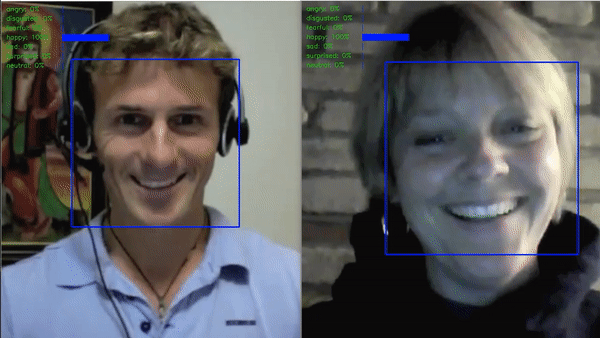
An Emotion AI designed for online healthcare coaching. Sample video source (left): YouTube.
This module, hosted on AWS, facilitates automated video processing and emotion analysis reporting. Upon video upload to a designated S3 bucket:
- An AWS EC2 instance is automatically triggered, initiating the AI analysis.
- The resultant EmotionAI report for each coaching session is automatically stored, either in S3 or a chosen database.
- A summary email detailing the findings is automatically sent to the designated coach or manager.
Illustrated below is the AI module’s operational workflow, accompanied by a cost estimation (referencing AWS’s 2018 pricing structure).
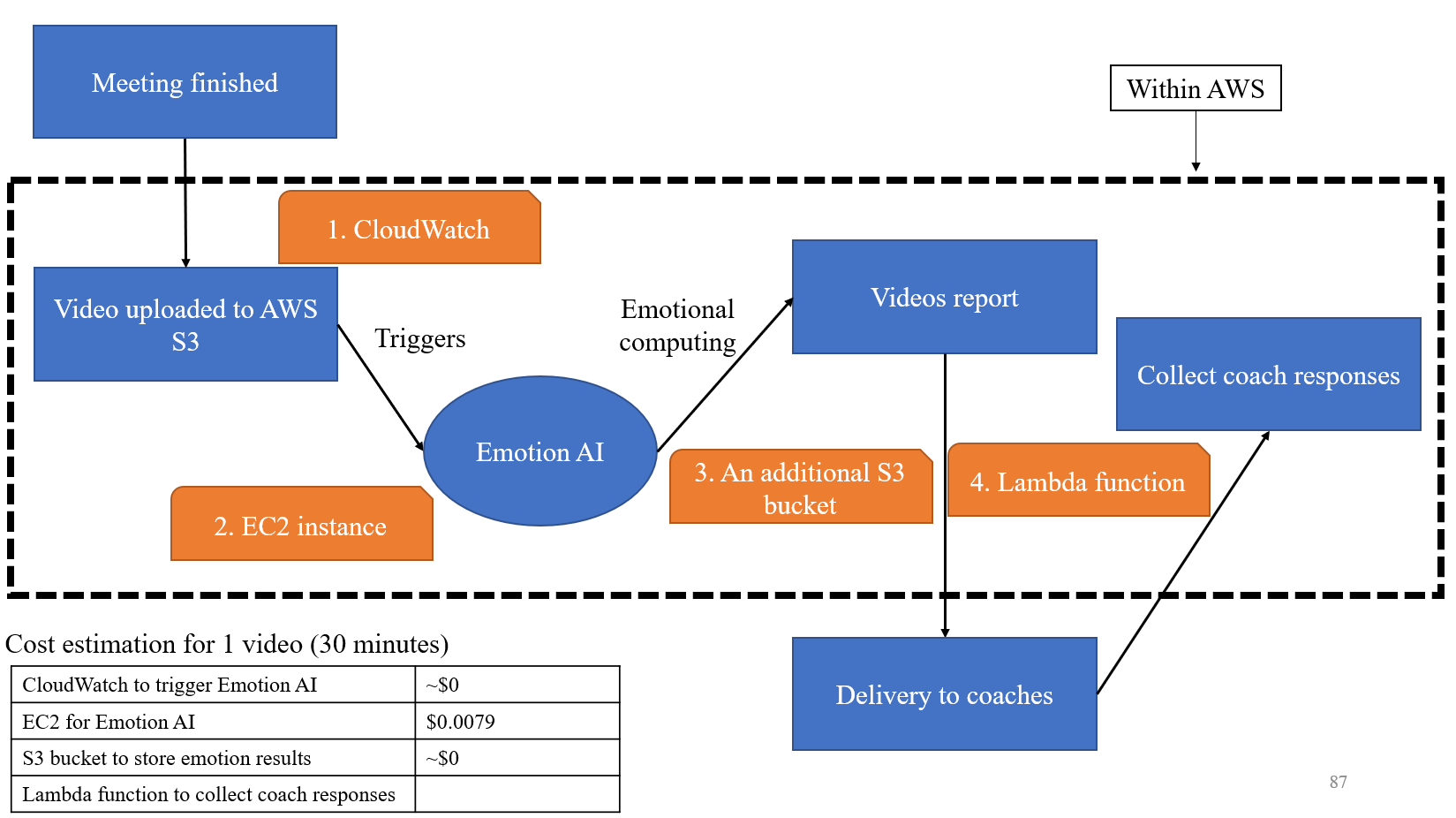
T5. Health Expenditure Prediction AI
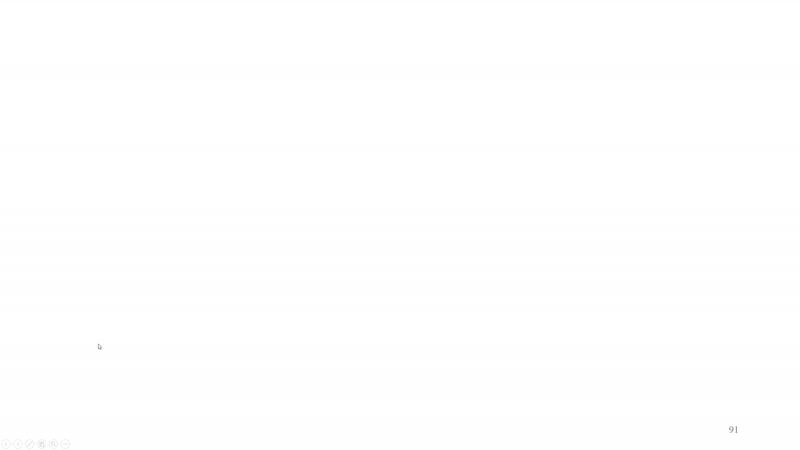
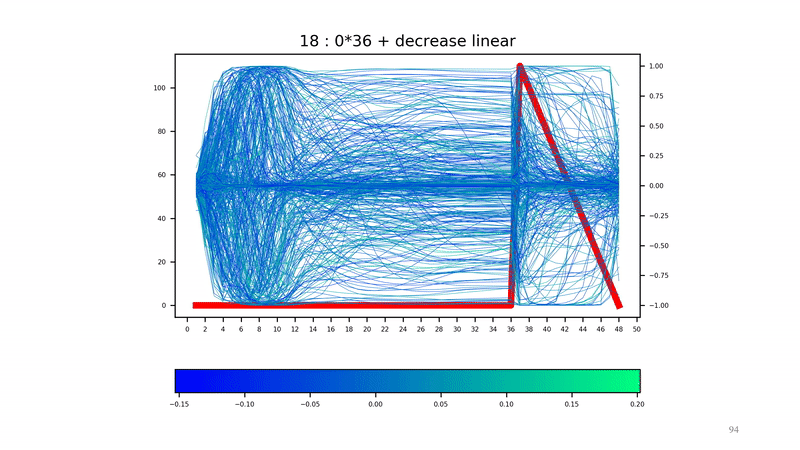
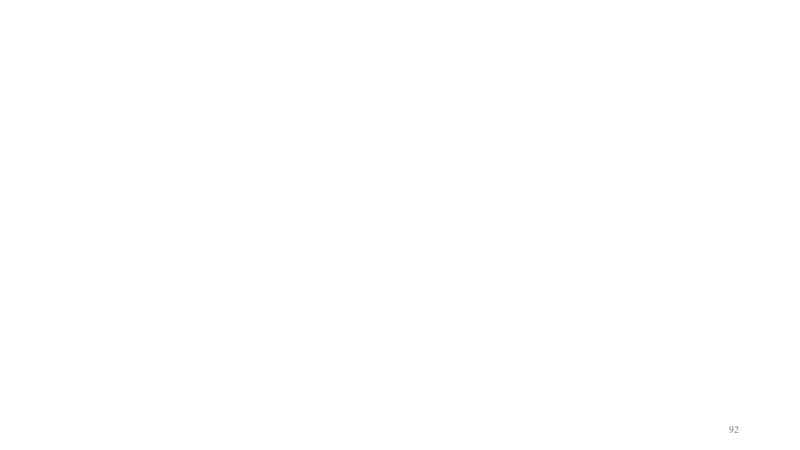
Weiguang Wang, Margrét V. Bjarnadóttir, and Gordon Gao, “How AI Plays Its Tricks: Interpreting the Superior Performance of Deep Learning in Cost Prediction.” https://papers.ssrn.com/sol3/papers.cfm?abstract_id=3274094
B1. AI and Worker Experience — Medical Coding AI
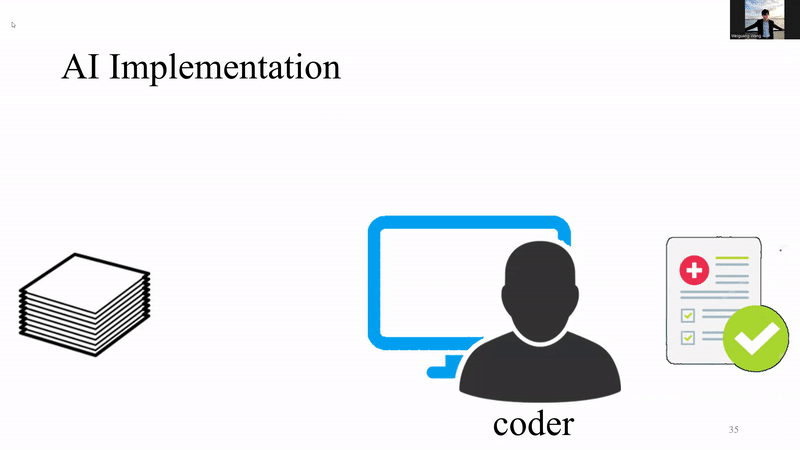
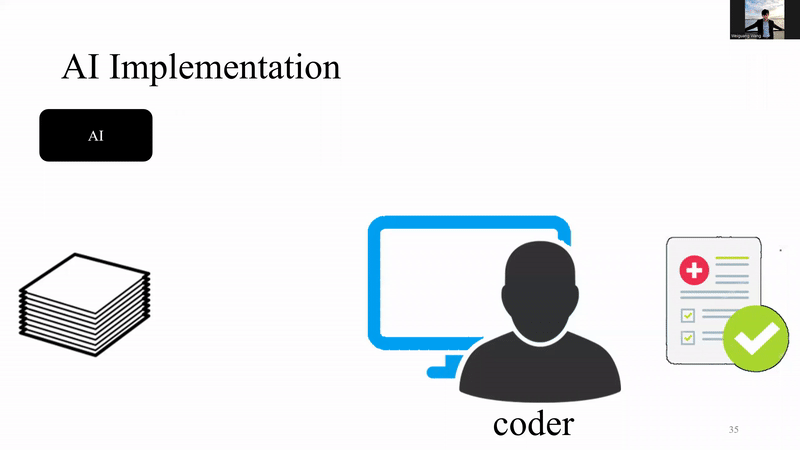
We designed and integrated an AI system for medical coding within clinical notes. While this enhances coder productivity, there is notable variability in user acceptance and resistance to the AI.
Weiguang Wang, Gordon Gao, and Ritu Agarwal, “Friend or Foe? The Influence of Artificial Intelligence on Human Performance in Medical Chart Coding” https://papers.ssrn.com/sol3/papers.cfm?abstract_id=3405759
Abstract
As AI applications become more pervasive, it is critical to understand how knowledge workers with different levels and types of experience can team with AI for productivity gains. We focus on the influence of two major types of human work experience, narrow experience based on the specific task volume and broad experience based on seniority, on the human-AI team dynamics. We developed an AI solution for medical chart coding in a publicly traded company and conducted a field study among the knowledge workers. Based on a detailed analysis performed at the medical chart level, we find evidence that AI benefits workers with greater task-based experience, but senior workers gain less from AI than their junior colleagues. Further investigation reveals that the relatively lower productivity lift from AI is not a result of seniority per se, but lower trust in AI, likely triggered by the senior workers’ broader job responsibilities. This study provides new empirical insights into the differential roles of worker experience in the collaborative dynamics between AI and knowledge workers, which have important societal and business implications.
B2. mHealth, C-Section Reduction, and Family
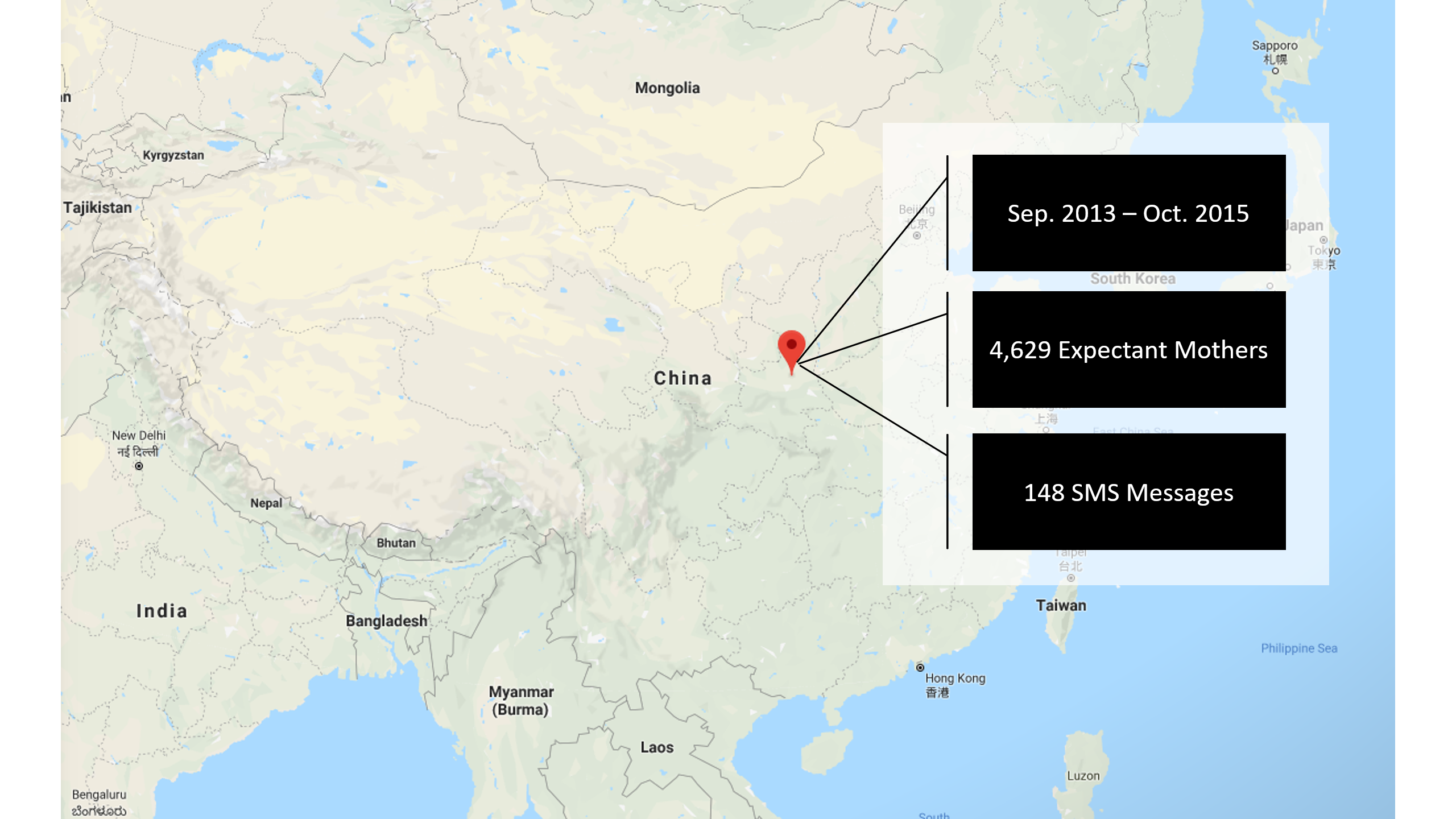
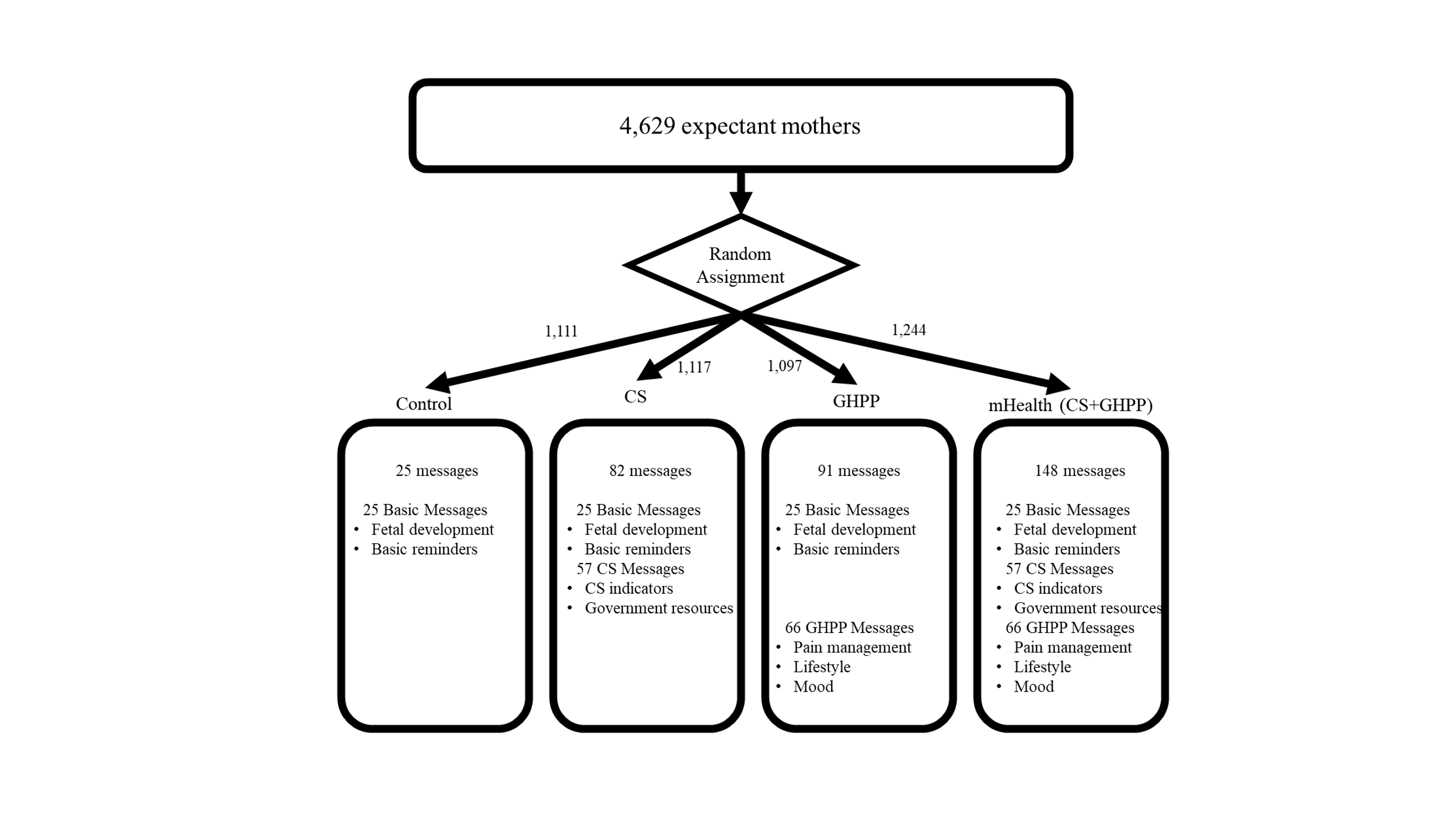
“How mHealth Helps Informationally Disadvantaged Mothers: The Role of Family Information Provision and Family Structure.” with Yanfang Su (co-first author), Gordon Gao, and Ritu Agarwal
Abstract
mHealth, the use of mobile technologies for healthcare management and delivery, offers great promise to promote health and improve care. However, to date, most mHealth treatments have failed to demonstrate a significant impact on clinical outcomes, and there is surprisingly little knowledge of factors that drive its efficacy. This study examines mHealth effectiveness by investigating both mHealth design and social support. To do so, we leverage one of the world’s largest field experiments on improving the health of expectant mothers and reducing the rate of cesarean sections. We hypothesize that 1) the combination of both self-directed mHealth and provider-directed mHealth ensures the highest mHealth effectiveness; 2) the husband, as one of the most significant social supports for expectant women, can be an important moderator of mHealth effectiveness. Our analyses show that the combined mHealth design achieves significant reduction in cesarean section use. In addition, a husband’s healthy behavior is pivotal in enabling mHealth interventions to be effective we find that the cesarean section reduction rate of women whose husbands engage in healthy behavior is four times higher than it is for those whose husbands do not fully engage in healthy behavior. Further analyses reveal that the husband’s healthy behavior has a stronger influence on mHealth effectiveness when the wife has higher status in the marriage. Our findings represent the first study to examine the effectiveness of these two mHealth designs (self-directed and provider-directed) and the critical role of social support in determining mHealth effectiveness. The study has important implications for both academic research and the practice of mHealth.
B3. Knowledge Trap in Human-AI Teaming
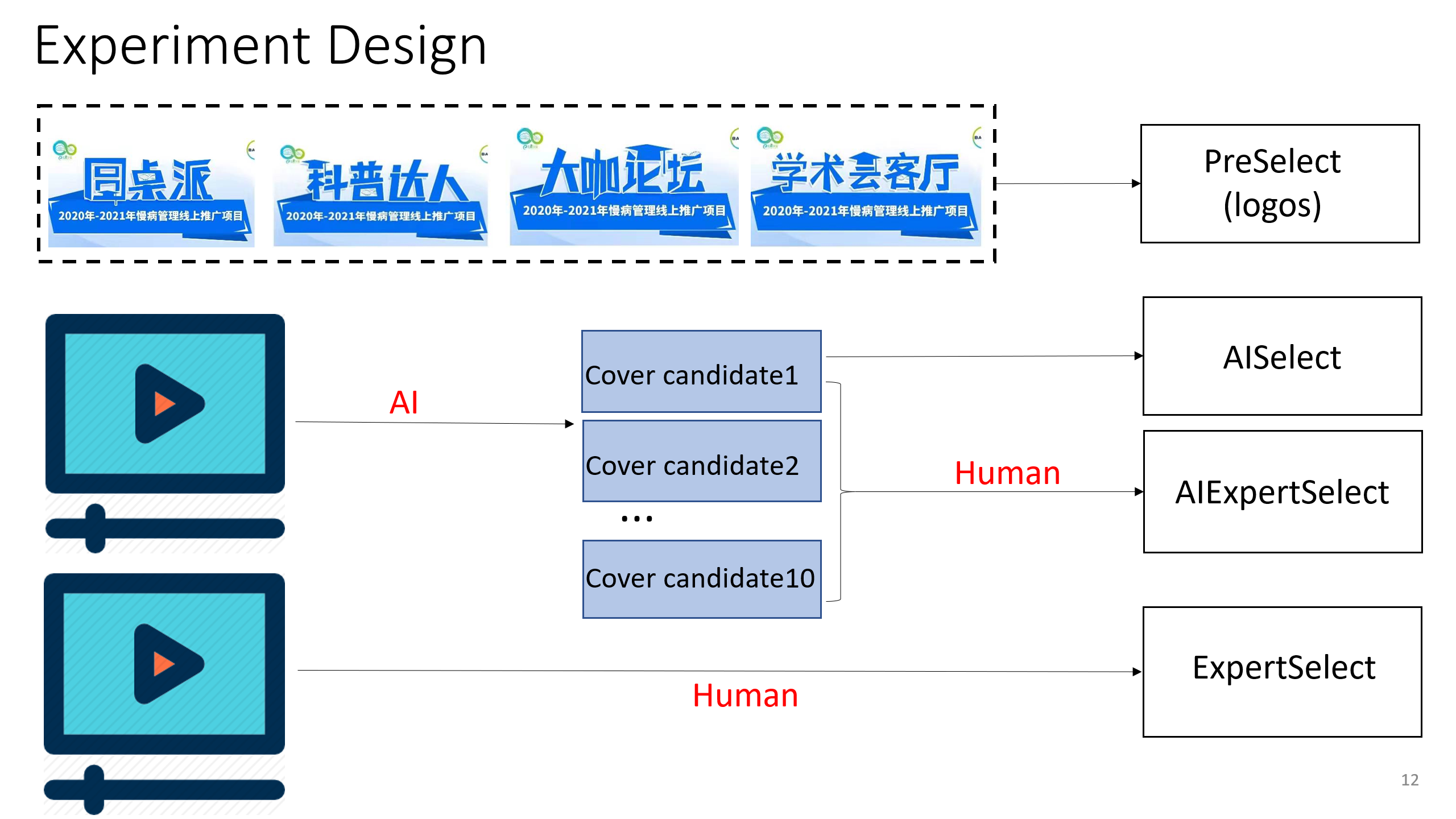
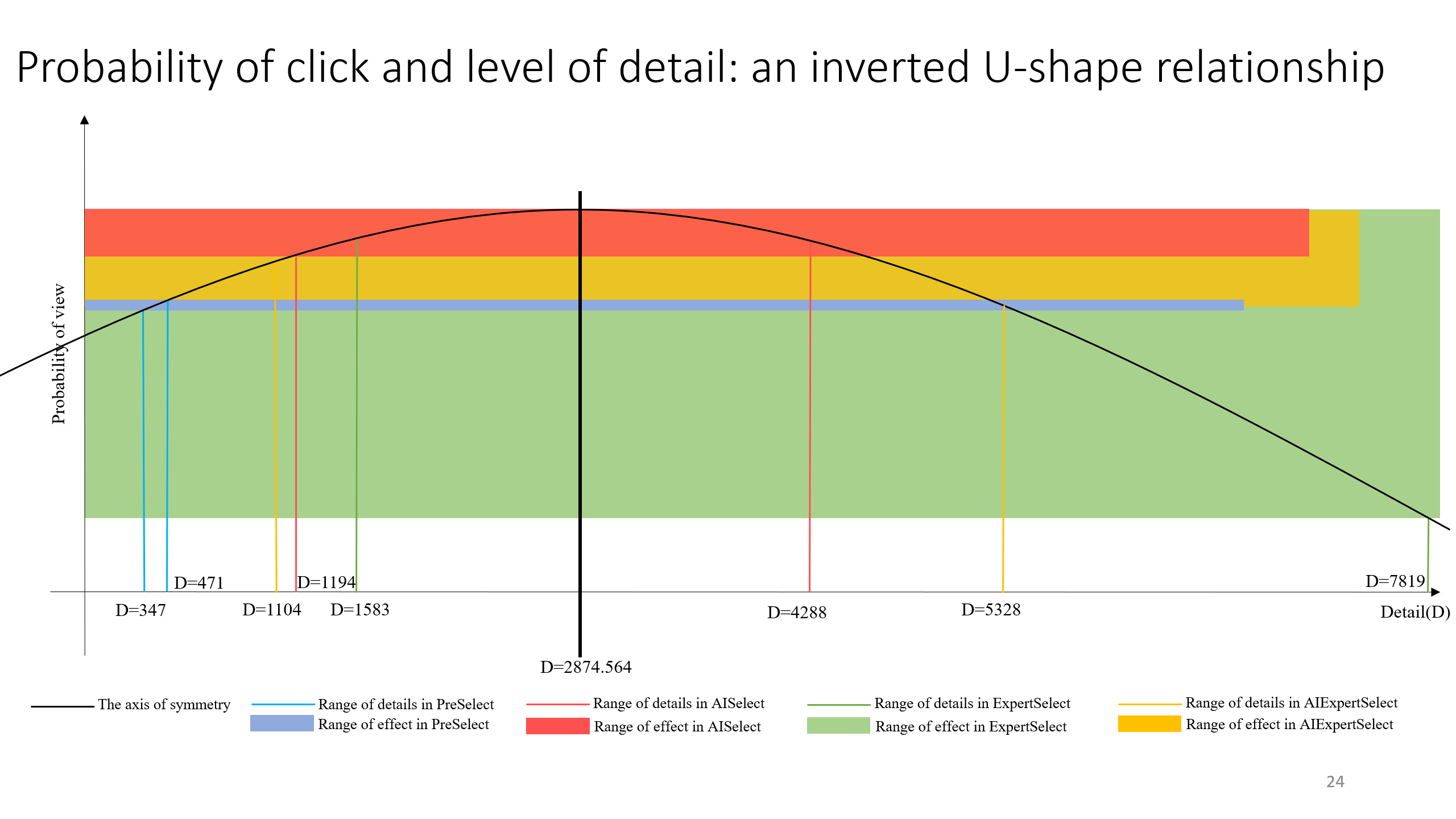
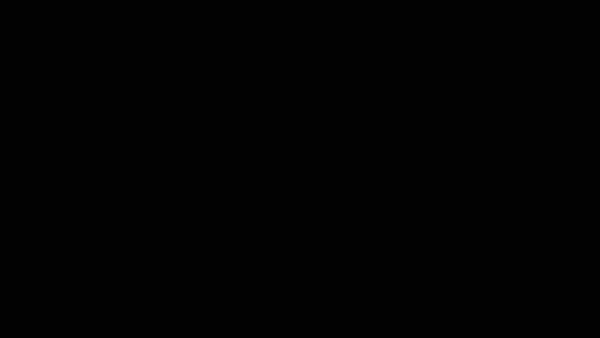
“Knowledge Trap: Human Experts Distracted by Details When Teaming with AI.” with Xiaopei Liu, Xi Zhang, and Yili (Kevin) Hong
Abstract
In the emerging literature on human–artificial intelligence (AI) teaming, the effectiveness of teaming is established on the complementarity between the unique advantages of both human experts and AI. Due to the nature of machine learning, contemporary AI can outperform human experts in narrow and well-defined tasks. However, human experts possess broad, context-aware, and forward-looking knowledge. While the entire human domain knowledge cannot be easily modeled, AI is positioned as augmented intelligence so that complex decision-making relies on human experts for final judgment. However, the broad knowledge of human experts is not always beneficial. We conduct a field experiment that showcases a phenomenon of knowledge trap in the context of human–AI teaming, in which human experts’ overfocus on details hurts their performance compared with AI’s concentrated yet narrow focus. Based on data from our field experiment, on average, video thumbnails selected by human experts attract fewer views than those selected by AI. Even more surprisingly, human and AI teaming together performs worse than just AI. This difference can be explained by the human experts’ overfocus on the thumbnail’s coverage of video details. While such details are helpful to certain subgroups, such as long videos and videos with less information in the title, we observe an inverted U-shaped relationship between the probability of a video being viewed and the level of details in its thumbnail. Therefore, compared with AI’s sole focus on visual features, domain expertise’s overemphasis on details becomes a knowledge trap, which largely reduces the effectiveness of human–AI teaming.
B4. Misinformed Clout
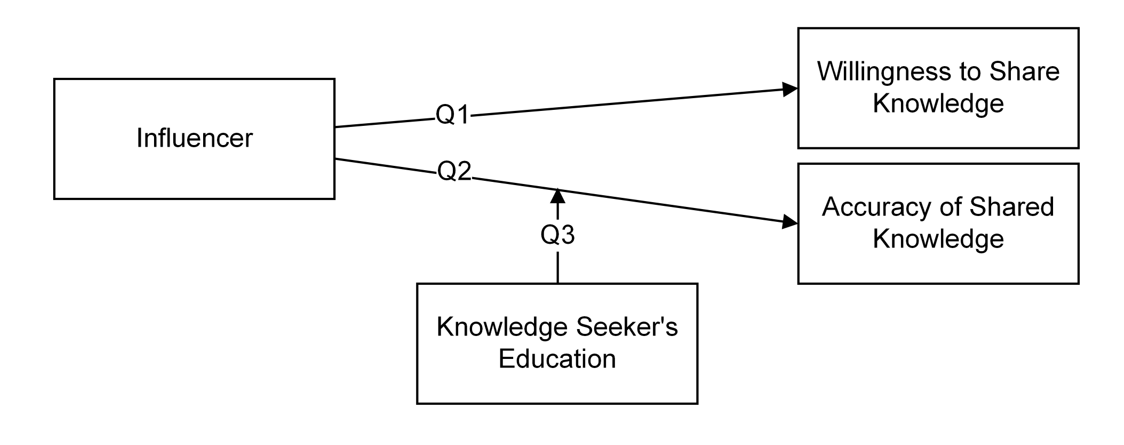
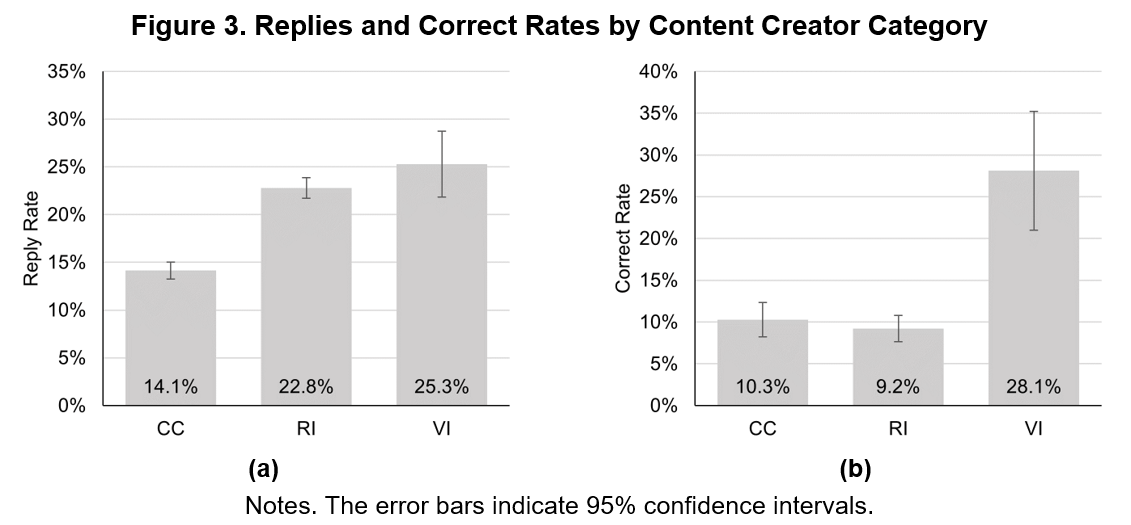
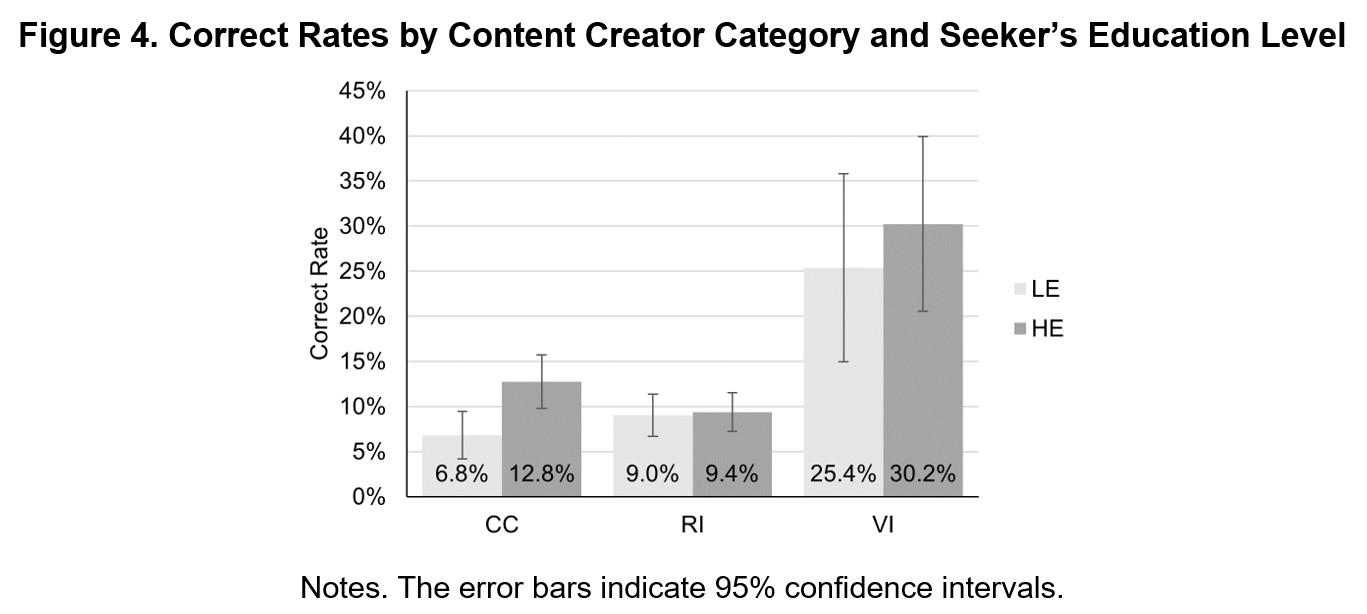
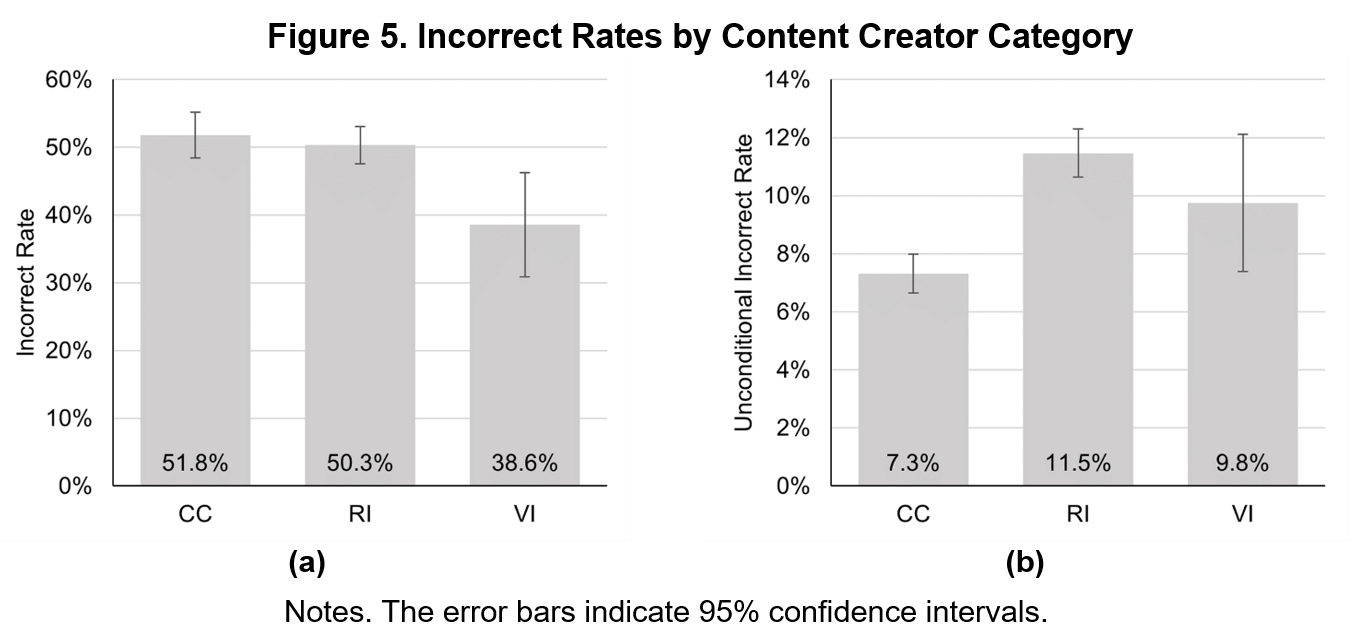
“Misinformed Clout: Evidence from a Field Experiment” with Ningning Cheng, Hongfei Li, and Yili (Kevin) Hong
Abstract
Online influencers are a group of socially engaged content creators who are widely perceived as domain experts and thus exert great social influence. In areas such as public health, it is crucial that the content shared by online influencers is scientifically accurate, given how much they are trusted and the extent to which they can influence others’ decisions. However, recent developments in content creation have decoupled influencers’ domain expertise from their social influence, leading to widespread misinformation. Whereas this jeopardizes both public interest and platform sustainability, little academic research has examined content creators’ expertise and influence. To fill this research gap, we conduct a large-scale field experiment on a large online fitness platform, examining the fitness influencers on this platform. Adopting a correspondence design, we programmed fictitious fitness users to send a fitness-related question via private messaging to both influencers and common content creators and analyze their responses. We find that while influencers are willing to share knowledge, they have surprisingly low correct rates. Particularly, influencers’ correct rates are indistinguishable from those of common content creators and even lower in some cases. We further explore how knowledge seekers’ education levels affect these correct rates. We observe that influencers are more prone to rejecting correct information from well-educated users and to providing wrong answers. These findings have critical implications for both academic research and platform operations, highlighting the need for greater scrutiny and regulation of online content creators.
B5. Family Colocation and Hurricane
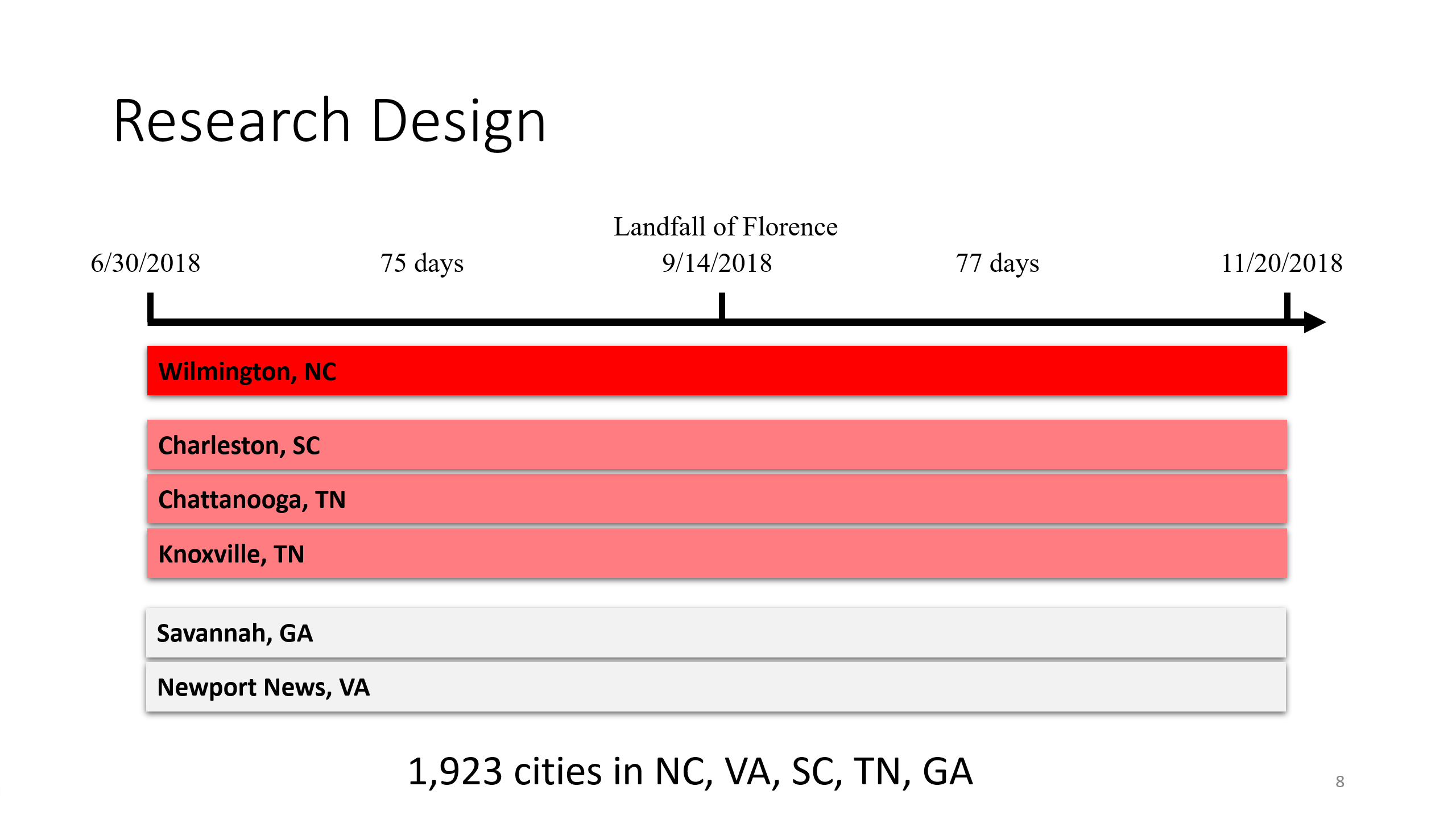
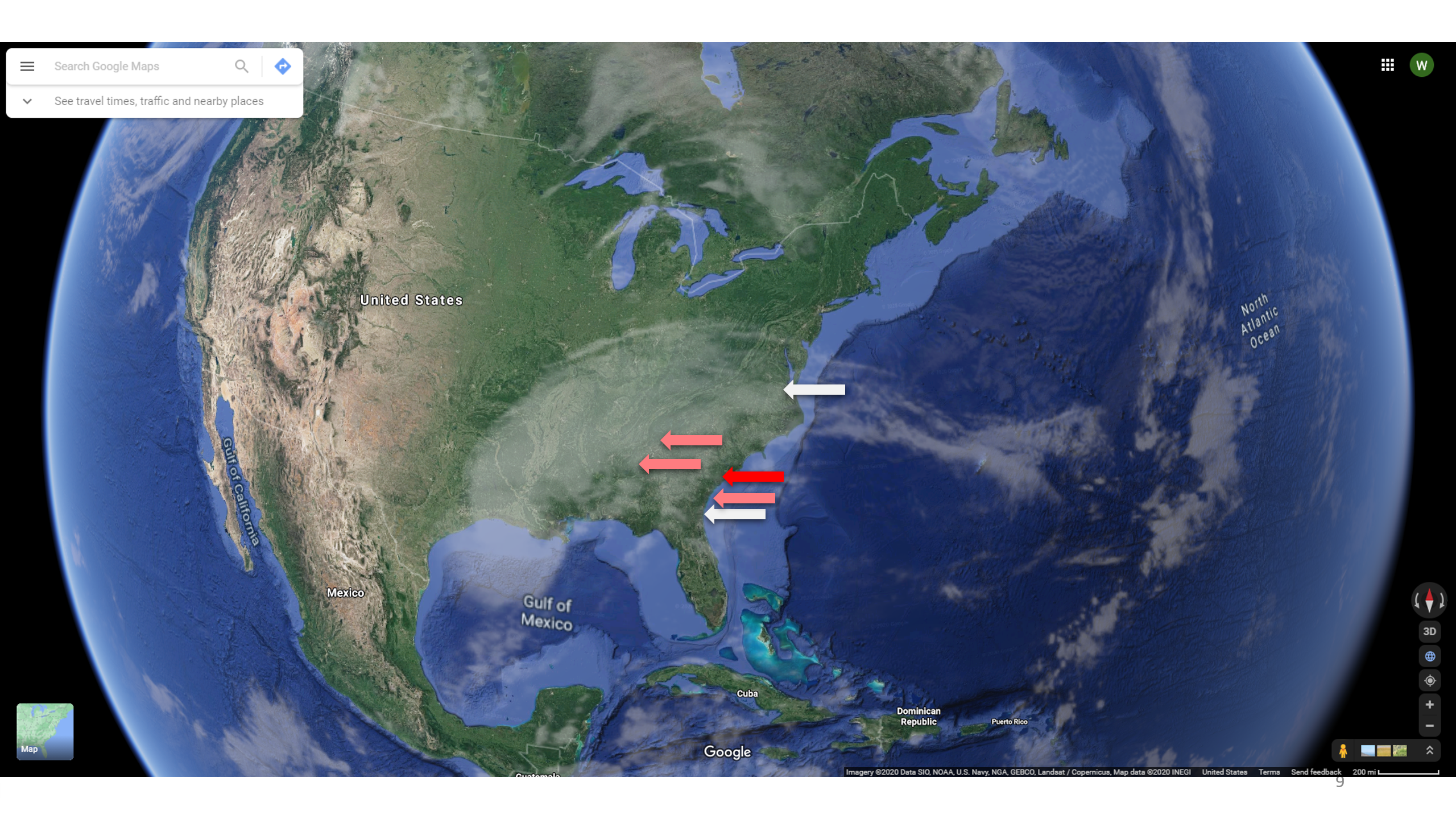
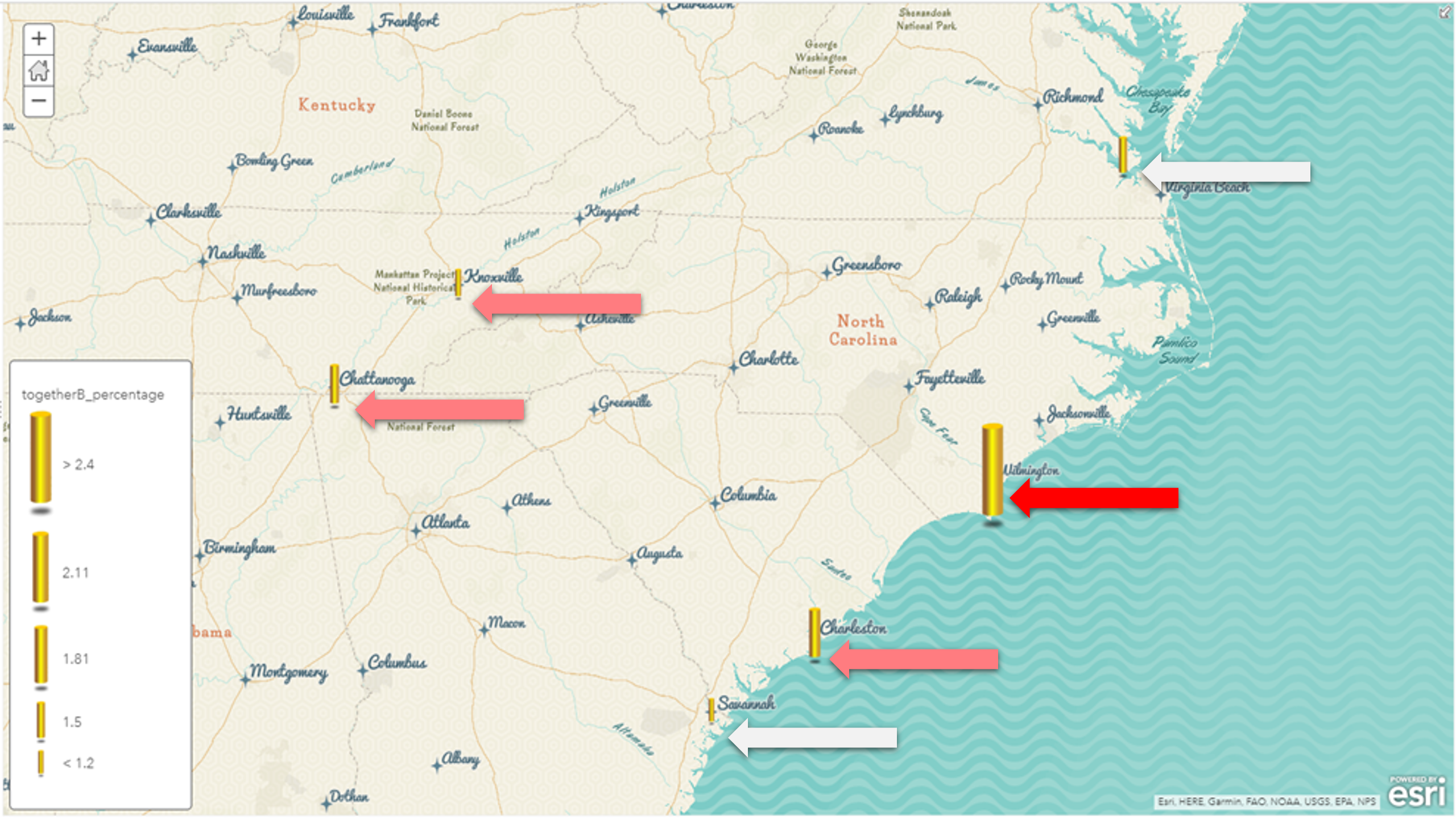
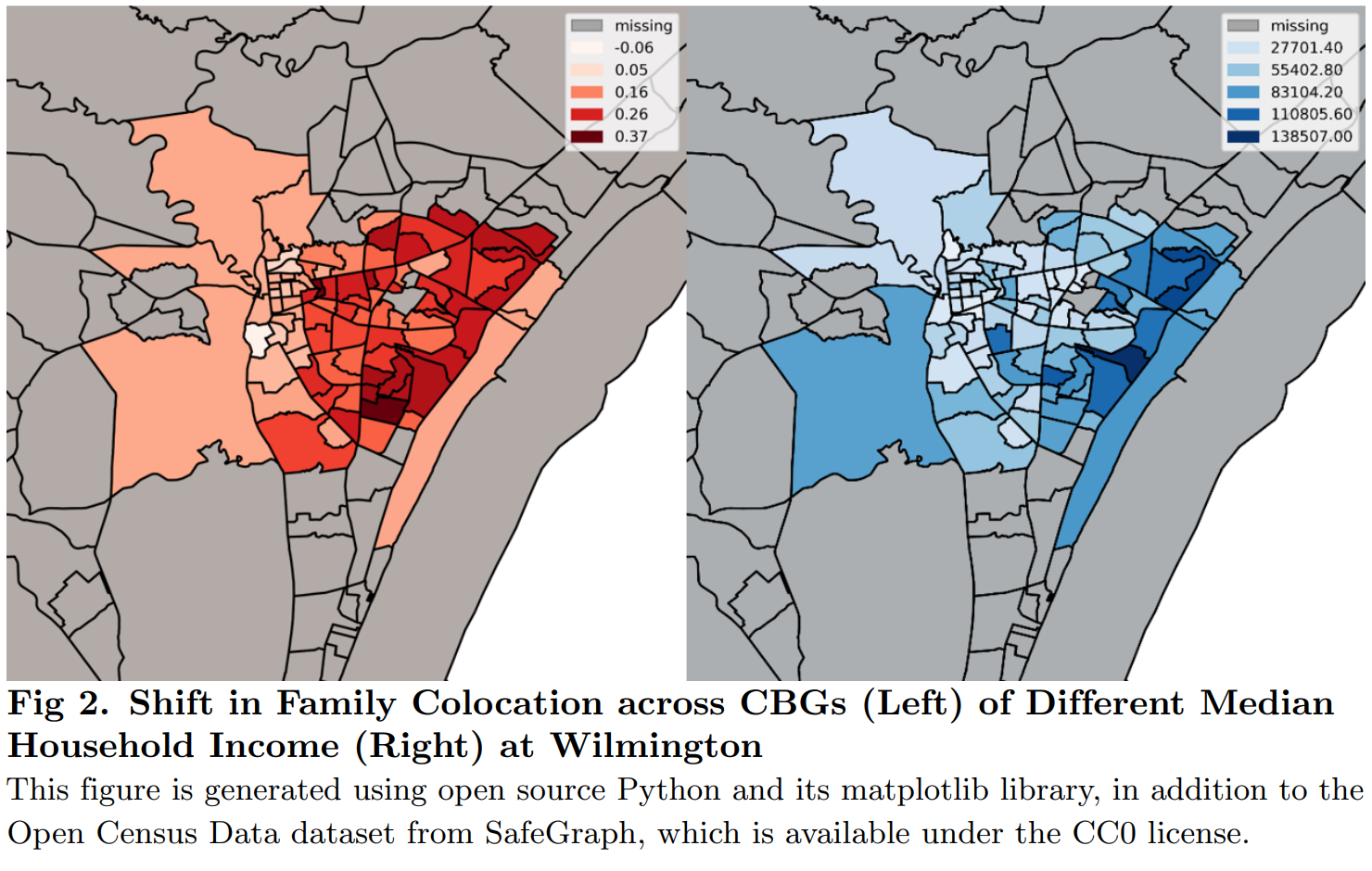
Weiguang Wang, Natasha Z Foutz, Guodong (Gordon) Gao, 2022, “Huddling with Families after Disaster: Human Resilience and Social Disparity.” PLOS One.
Abstract
Disasters, from hurricanes to pandemics, tremendously impact human lives and behaviors. Physical closeness with family post-disaster plays a critical role in mental healing and societal sustainability. Nonetheless, little is known about whether and how family colocation alters after a disaster, a topic of immense importance to a post-disaster society. We analyze 1 billion records of population-scale, granular, individual-level mobile location data to quantify family colocation, and examine the magnitude, dynamics, duration, and socioeconomic heterogeneity of the shift in family colocation from the pre- to post-disaster period. Leveraging Hurricane Florence as a natural experiment, and Geographic Information System (GIS), machine learning, and statistical methods to investigate the shift across the landfall (treated) city of Wilmington, three partially treated cites on the hurricane’s path, and two control cities off the path, we uncover dramatic (18.9%), widespread (even across partially treated cities), and enduring (over 3 months) escalations in family colocation. These findings reveal the powerful psychological and behavioral impact of the disaster upon broader populations, and simultaneously remarkable human resilience via behavioral adaptation during disastrous times. Importantly, the disaster created a gap across socioeconomic groups non-existent beforehand, with the disadvantaged displaying weaker lifts in family colocation. This sheds important lights on policy-making and communication to promote sustainable family colocation, healthy coping strategies against traumatic experiences, social parity, and societal recovery.